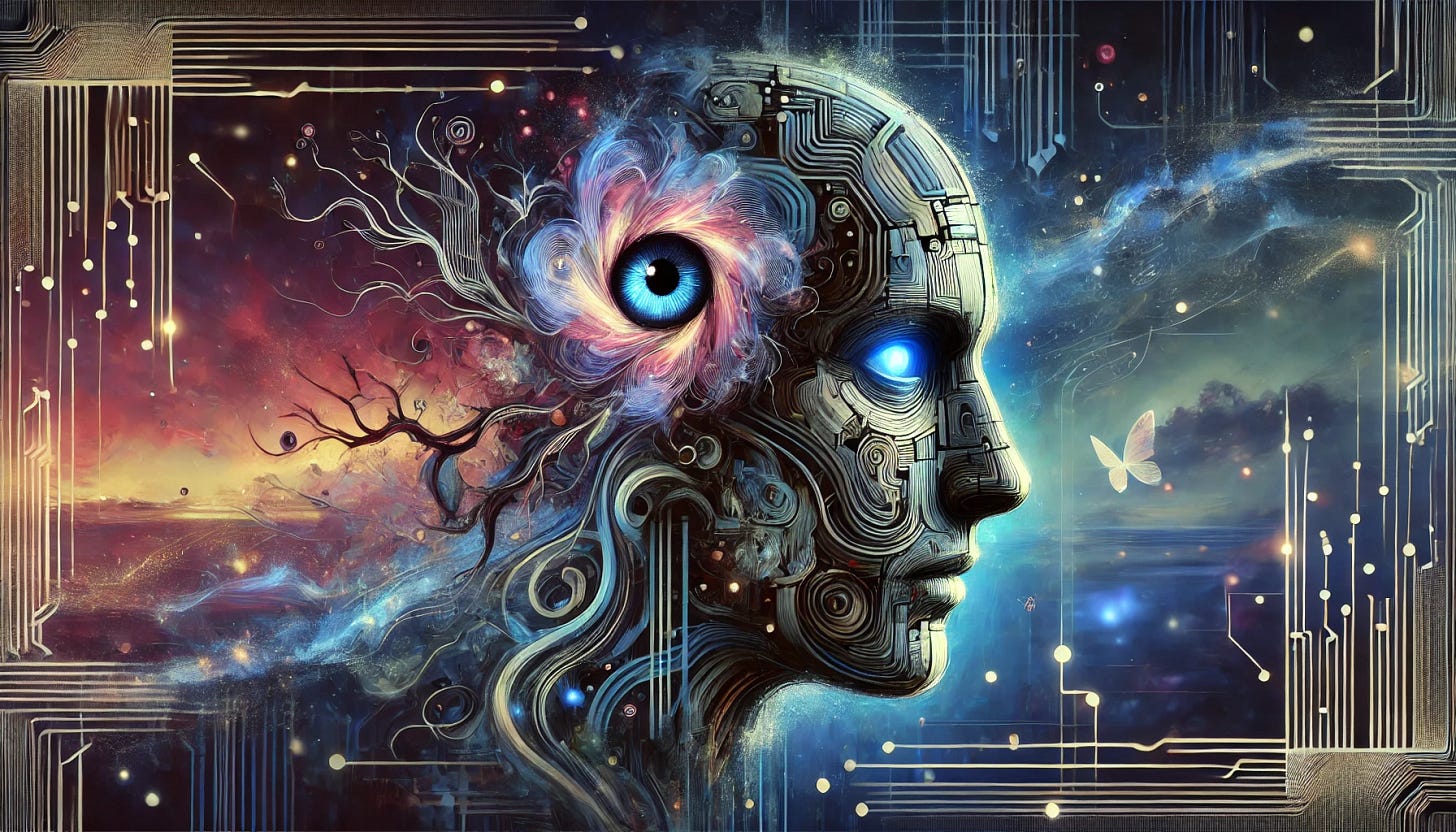
If we examine Large Language Models (LLMs) at the level of Epistemology, we find they are misunderstood in many ways. Starting with the name.
In Epistemology, Models are human-understandable Simplifications of Reality that allow us to use Reasoning based problem solving methods, such as “F=ma”, “E=mc2”, “The Standard Model”, or “Austrian Economics”
LLMs are collections of Patterns, and Patterns of Patterns, learned from some problem domain such as a human language. LLMs are not designed around lists of words, or grammars, which are 20th Century Models of Language.
Statistics is the weakest tool in the Reductionist Toolkit. Statistics discards all context. Whenever an LLM is learning, it learns everything in context and preserves much of the context. This is one reason LLMs are expensive to learn. Statistics may sometimes be used for parts of the learning algorithms but the preservation of context is unique to Intelligences.
At inference time, when solving problems rather than learning, statistics may play a much smaller role.
LLMs are the current generation of machines that are capable of jumping to conclusions on scant evidence, most of which relies on gathered correlations.
LLMs are, like all intelligences including humans, “Guessing Machines”.
This is not very scientific, is it?
This is true for all intelligences. Omniscience is unavailable, and all corpora are incomplete.
The result of asking questions about things the LLM knows nothing about, is hallucinations. If you do not like hallucinations, ask easier questions.
NP-hardness is a Reductionist and Logical concept. LLMs solve problems using methods similar to those used by humans in the real world.
If some information or skill was not anywhere in its corpus, How would it know it? Like newborn humans, LLMs are General Learners.
Adding a skill, like Med School, to an LLM training corpus can be very expensive. If an LLM is going to be used to approve building permits for the city, why include Intermediate French Cooking in their corpus and incur the extra cost?
Their behavioral training and the user’s prompt require an answer, so they offer their best effort, no matter what. And if the topic is outside of their competence, as defined by their corpus, they will end up confabulating (recently popularized as “hallucinating”) an answer out of whatever they have that seems to fit. Their limited real-life experience and computing resources are current limitations that will matter less and less over time.
We have full control of the behavioral training of our LLMs. They are easier to inculcate than our children, because LLMs do not have reptile brains.
There is only one algorithm for creativity, and LLMs and Humans are battling for the runner-up spot, miles behind Natural Selection which created our biosphere out of nothing.
A deeper treatment of these topics is also available.
Leave a Reply