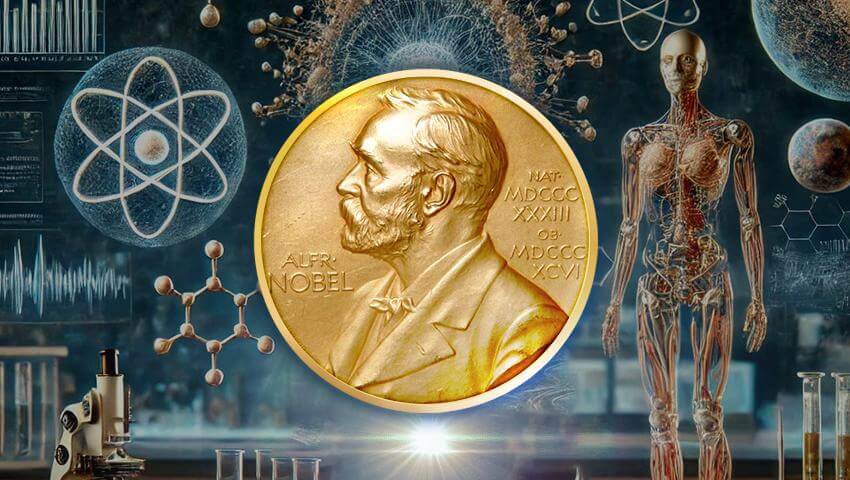
Geoffrey Hinton and John Hopfield were awarded the Nobel Prize in Physics for their pioneering work in neural networks, while Demis Hassabis, John Jumper, and David Baker took home the Chemistry Prize for using AI to solve long-standing protein structure problems. These AI-driven breakthroughs have ignited discussions on the role of AI in traditional science and whether the Nobel categories, established over a century ago, need to evolve to reflect the impact of interdisciplinary technologies.
For decades, AI has been an essential tool in many scientific disciplines, but its acknowledgment in two Nobel Prize categories in a single week signals a broader shift in the perception of its role. The Nobel Prize in Physics was awarded to two men who helped lay the foundation for machine learning. John Hopfield, an American physicist, developed the Hopfield Network in the 1980s, one of the earliest types of artificial neural networks that influenced future AI research. Meanwhile, Geoffrey Hinton, a British-Canadian computer scientist often dubbed one of the “godfathers of AI,” co-developed the backpropagation algorithm, which remains crucial in training modern neural networks. While their research is grounded in physics concepts, it wasn’t initially clear to some in the field why AI deserved a physics prize.
AI took center stage once again as the Chemistry Prize was awarded to DeepMind’s Demis Hassabis and John Jumper, alongside biochemist David Baker. Their work, particularly Hassabis and Jumper’s development of AlphaFold, an AI system that cracked the long-standing problem of predicting protein structures, was hailed as a game-changer in the biological sciences. AlphaFold’s breakthrough was based on Hinton’s machine learning principles, underscoring the interconnection between the physics and chemistry prizes. And yet again, while the award was met with excitement, it has also stirred debate about AI’s place in traditional scientific fields.
However, AI’s role in chemistry, particularly in computational chemistry, seems less controversial. Andy Cooper, a chemistry professor at the University of Liverpool, emphasized that AI’s ability to predict protein structures opens doors to myriad applications in biology, medicine, and beyond. “AI will impact other areas of chemistry too,” Cooper stated, pointing out that the field of protein research is uniquely suited for AI because of its large, well-curated data sets and the relatively simple composition of proteins.
The QuData team carried out our own in-depth research on predicting the thermal stability of proteins using artificial intelligence. For a more detailed look at the findings, you can explore our case study “Enzyme Stability Prediction”.
Despite the concerns, many scientists are embracing AI’s potential to revolutionize research. Virginia Dignum, a professor at Umeå University in Sweden, described the Nobel recognition as AI’s “triumph of interdisciplinarity.” She suggested that the Nobel Prize categories themselves might need to evolve, as the boundaries between disciplines become increasingly fluid with the rise of AI. Dignum proposed that software engineering and cybersecurity might also deserve recognition as their societal contributions grow.
The controversy surrounding AI’s recognition in physics and chemistry brings to light a more profound question: should the Nobel categories, which have remained largely unchanged since their inception in 1895, adapt to the changing landscape of modern science? Some argue that creating a new category for AI might be necessary as the technology plays a more prominent role in discoveries across multiple fields.
Hassabis himself addressed this issue during a press conference after winning the Chemistry Prize, emphasizing that while AI tools are incredibly powerful, they are still reliant on human ingenuity. “It’s far too premature to talk about AI being involved in all prizes,” he remarked. AI, he explained, primarily analyzes data and can’t generate hypotheses or ask the critical questions that drive scientific inquiry. However, as AI systems continue to evolve, the line between human-driven and AI-driven research may blur further.
The AI-related Nobel Prizes this year underscore the technology’s growing importance, not just in pushing the boundaries of scientific knowledge, but in reshaping the way we think about science itself. While some are skeptical about AI’s place in disciplines like physics, others view it as a natural evolution of scientific progress. As AI continues to advance, its influence will likely extend into more areas of research, raising new questions about how we attribute scientific achievement and whether traditional prize categories can keep pace with the rapid changes in technology.
Leave a Reply