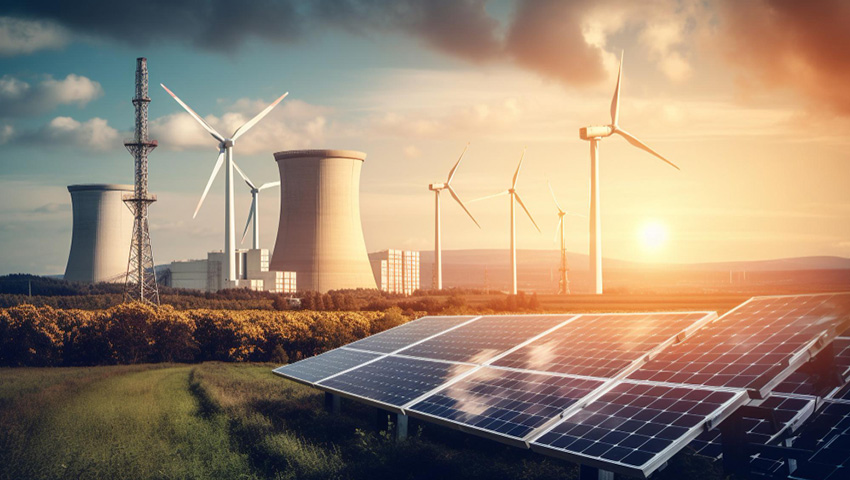
Amidst the fervor to advance AI capabilities, Lincoln Laboratory has dedicated efforts to curtail AI models’ energy consumption. This pursuit aims to foster efficient training methods, reduce power usage, and introduce transparency in energy consumption.
The aviation industry has begun presenting carbon-emission estimates for flights during online searches, encouraging users to consider environmental impact. However, such transparency is yet to permeate the computing sector, where AI models’ energy consumption surpasses that of the entire airline industry. The burgeoning size of AI models, exemplified by ChatGPT, indicates a trajectory toward larger-scale AI, foretelling data centers consuming up to 21% of global electricity by 2030.
The MIT Lincoln Laboratory Supercomputing Center (LLSC) has taken innovative strides in curtailing energy usage. They’ve explored various approaches, from power-capping hardware to terminating AI training early without compromising model performance significantly. Their objective is not just energy efficiency but also driving transparency in the field.
One avenue of LLSC’s research focuses on power limitations of graphics processing units (GPU). By studying power caps’ effects, they’ve noted a 12-15% reduction in energy consumption while extending task completion times by a negligible 3%. Implementing this intervention across their systems led to cooler GPU operations, promoting stability and longevity while decreasing stress on cooling systems.
Furthermore, LLSC has crafted software integrating power-capping capacities into the widely used scheduler system, Slurm, enabling users to set limits across the system or per job basis effortlessly.
Their initiatives transcend mere energy conservation, branching into practical considerations. LLSC’s approach not only saves energy but also diminishes the center’s embodied carbon footprint, delaying hardware replacements and reducing overall environmental impact. Their strategic job scheduling also minimizes cooling requirements by running tasks during off-peak times.
Collaborating with Northeastern University, LLSC introduced a comprehensive framework for analyzing high-performance computing systems’ carbon footprint. This initiative enables practitioners to evaluate system sustainability and plan modifications for future systems effectively.
Efforts extend beyond data center operations, delving into AI model development. LLSC is exploring ways to optimize hyperparameter configurations, predicting model performance early in the training phase to curtail energy-intensive trial-and-error processes.
Moreover, LLSC has devised an optimizer, in partnership with Northeastern University, to select the most energy-efficient hardware combinations for model inference, potentially reducing energy usage by 10-20%.
Despite these strides, challenges persist in fostering a greener computing ecosystem. The team advocates for broader industry adoption of energy-efficient practices and transparency in reporting energy consumption. By making energy-aware computing tools available, LLSC empowers developers and data centers to make informed decisions and reduce their carbon footprint.
Their ongoing work emphasizes the need for ethical considerations in AI’s environmental impact. LLSC’s pioneering initiatives pave the way for a more conscientious and energy-efficient AI landscape, driving the conversation toward sustainable computing practices.